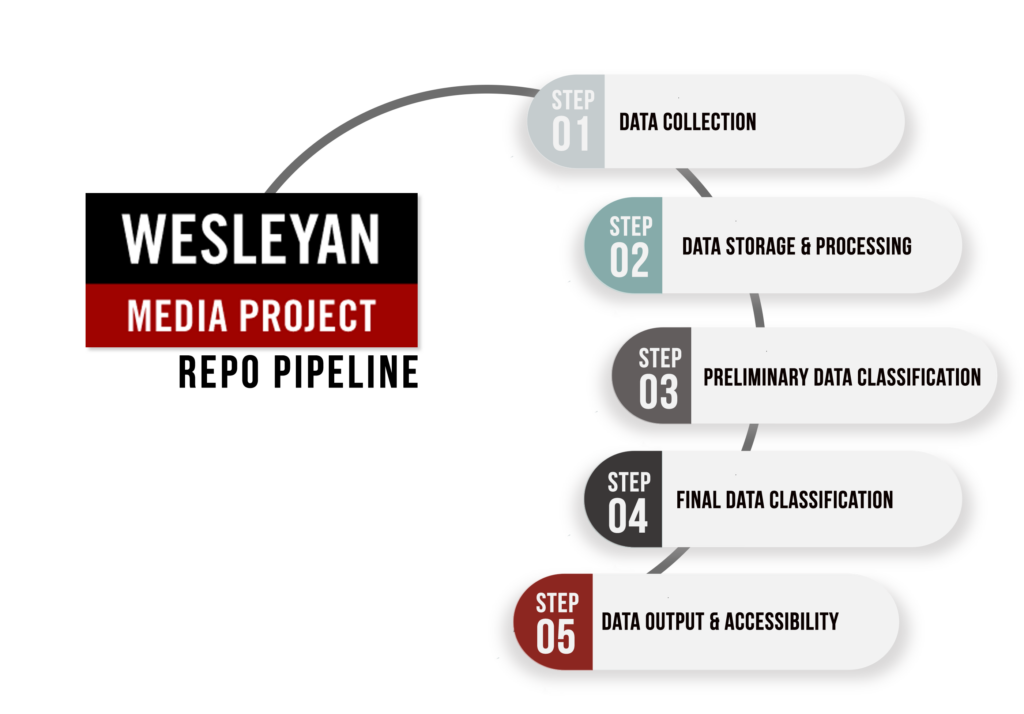
Our GitHub repositories include four categories: Data Collection and Storage, Data Processing, Data Classification, Data Output & Accessibility.
Text, audio, and image are extracted from the ads in order to use these three different components for machine learning and entity linking. This allows us to find similar digital ads across platforms based on the ads’ likeness in either component.
For each ad that is found, a unique ad identification number is created. Depending on which the ad includes, image ids, videos files, and text are saved for each unique ad id. Next, we clean the raw data with deduplication and then train this cleaned data for each type of element it includes. Text is processed with OCR, video is processed with ASR, and image is processed with facial recognition.
The classification step aims to take the different elements of an ad and organize the data to a classifier of interest like party (i.e., which party is the sponsor), race of focus (which federal race is the ad related to), ad tone (positive, negative, or contrasting), or goals (i.e., fund-raising) and policy issues.
For those interested only in the final data product, see Data Output & Accessibility[add link]. For those interested in the full data collection and processing steps, start with Data Collection and Storage[add link].
NOTE: This project is under construction. Our Figshare datasets are not yet public.